USA
Big Data Trends to Consider in 2021
Big Data is keeping up with the pace. According to some studies, there are 40 times more bytes in the world than there are stars in the observable universe. There is simply an unimaginable amount of data being produced by billions of people every single day. The global market size predictions prove it beyond any doubt.
It’s not a question of if you will use Big Data in your daily business routine, it’s when you’re going to start using it (if somehow you haven’t yet). Big Data is here and it’s here to stay for the foreseeable future.
For the last ten years, data volume is growing at a blistering pace. As more companies are operating bigger data volumes and rapidly developing the Internet of Things market, data volume will become even bigger next year.
What happens every minute (via Internet Live Stats):
- 6,123 TB Traffic produced by users
- 185,000,000 E-mails sent
- 5,200,000 Google searches
- 305,000 Skype calls
- 84,000 Instagram photos uploaded
Investigating demands in the market and keeping our finger on the pulse, we’ve prepared a brief overview of trends that you should definitely keep an eye on during 2021 if you’re into Big Data.
Knowing that the Big Data market is constantly evolving to meet customer demand, the 2020 predictions by Gartner are still on target for 2021.
1. Augmented Analytics
Augmented Analytics extends BI toolkit with AI and Machine Learning tools and frameworks.
This emerges from traditional BI where the IT department drives all tools. Self-service BI provides visual-based analytics for a business user and, in some cases, for an end-user. Augmented Analytics is the next evolutionary step of self-service BI. It integrates Machine Learning and AI elements into a company’s data preparation, analytics, and BI processes to improve data management performance.
Augmented Analytics can reduce time-related to data preparation and cleaning. Creating insights for business people with little to no supervision takes up a large part of the day-to-day data scientists life.
Based on: Gartner
2. Continuous Intelligence
Continuous Intelligence is a process of integration of real-time analytics into current business operations.
According to Gartner, more than half of new major business systems will make business decisions based on real-time analytics by 2022. By integrating real-time analytics into business operations and processing current and historical data, continuous intelligence helps augment human decision-making as soon as new data arrives.
Based on: Gartner
Many organizations still only rely on historical and outdated data. Such organizations probably will fall behind in rapidly changing environments. So an organization should have a picture of its data constantly and immediately. Such data will boost the speed of issue identification and resolution and important decision-making.
Based on: Sumo Logic
3. DataOps
DataOps is similar to DevOps practices in direction, but is aimed at different processes.
Unlike DevOps, it collaborates practices towards data integration and data quality across the organization. DataOps focuses on reducing the end-to-end cycle of data starting from data ingestion, preparation, analytics and ends with chart creation, reports, and insights.
DataOps tackles data processing zones for employees who are less familiar with data flow. This is so people can focus more on domain expertise and less on how data runs through an organization.
Based on: Nexla
3.1 Rise of Serverless
With the strong presence of cloud solutions in the market, new trends and practices are emerging and intersect with each other. DataOps practices are designed to simplify and accelerate data flow, even by removing and improving organizational infrastructure. That’s why the DataOps toolkit contains so-called “Serverless” practices. Such practices allow organizations to reduce their amount of hardware, scale easily and quickly, and speed up data flow changes by managing data pipeline parts in the cloud infrastructure.
Based on: Forrester Global Business Technographics Developer Survey,
Serverless Technology Semiannual Report by New Relic
3.2 One step further: DataOps-as-a-Service
Implementing integration, reliability, and delivery of your data takes a lot of effort and skill. It takes Data Engineers, Data Scientists, and DevOps time to implement all DataOps practices. New products constantly appear on the market, and these products can implement these practices with your data.
These products provide a variety of DataOps practices that are pluggable, extendable and allow for the development of sophisticated data flows based on your data and also provide API for your Data Science department.
4. In-Memory Computation
In-Memory Computation is another approach for speeding up analytics.
Besides real-time data processing, it eliminates slow data access (disks) and bases all process flow entirely on data stored in RAM. This results in data being processed and queried at a rate more than 100 times faster than any other solution, which helps businesses make decisions and take actions immediately.
5. Edge Computing
Edge Computing is a distributed computing framework that brings computations near the source of the data where it is needed.
With increasing volumes of data that are transferred to cloud analytics solutions, questions arise as to the latency and scalability of raw data and processing speed. An Edge Computing approach allows for the reduction of latency between data producers and data processing layers and the reduction of the pressure on the cloud layer by shifting parts of the data processing pipeline closer to the origin (sensors, IoT devices).
Gartner estimates that by 2025, 75% of data will be processed outside the traditional data center or cloud.
Based on: MarketandMarkets, Gartner forecast
6. Data Governance
Data Governance is a collection of practices and processes that ensure the efficient use of information within an organization.
Security data breaches and the introduction of GDPR have forced companies to pay more attention to data. New roles have started to emerge like Chief Data Officer (CDO) and Chief Protection Officer (CPO) whose responsibility is to manage data under regulation and security policies. Data Governance is not only about security and regulations, but also about availability, usability, and the integrity of the data used by an enterprise.
Based on: Data Governance Market report by MarketandMarkets
Rapidly increasing growth in data volume, rising regulatory and compliance mandates are behind the massive growth in the global data governance market.
7. Data Virtualization
Data Virtualization integrates all enterprise data siloed across different systems, manages the unified data for centralized security and governance, and delivers it to business users in real time.
When different sources of data are used, such as from a data warehouse, cloud storage or a secured SQL database, a need emerges to combine or analyze data from these various sources in order to make insights or business decisions based on analytics. This is unlike the ETL approach that mostly replicates data from other sources. Data Virtualization directly addresses the data source and analyzes it without duplicating it in the data warehouse. This saves data processing storage space and time.
Based on: Gartner Market Guide for Data Virtualization,
ReportLinker
8. Hadoop > Spark
Market demands are always evolving and so are the tools. In modern data processing more and more engineering trends are affected by Big Data infrastructure. One of the notable software trends is migration into the cloud. So we can see how data processing is moving away from on-premise or data centers into cloud providers using AWS service for data ingestion, analytics, and storage.
With such shifts, not all tools are able to keep up with the pace. For example, most Hadoop providers still only support data center infrastructure, while frameworks like Spark feel very comfortable both in data centers and in the cloud. Spark is constantly evolving and progressing rapidly head-to-head with market demands giving more options for businesses like hybrid- and multi-cloud setup.
Based on: spark.apache.org/
Conclusion
Based on market projections, Big Data will continue to grow. According to several studies and forecasts its global market size will reach a staggering $250 billion by 2025.
Some trends from previous years such as Augmented Analytics, In-Memory Computation, Data Virtualization, and Big Data processing frameworks are still relevant and will have a great impact on business. For example, In-Memory Computation works more than 100 times faster than any other solution. This helps businesses make decisions and take actions almost instantly. As for Data Virtualization, which helps to save data processing storage space and time, almost 2/3rds of all companies will have already implemented this approach by 2022.
New trends are emerging as well. Such powerful tools as Continuous Intelligence, Edge Computing, and DataOps can help improve business and make things happen faster. For instance, Continuous Intelligence takes both historical data and real-time data into account. This significantly affects the way organizations make decisions and how efficient and fast they are. By 2022, more than 50% of new major business systems will make business decisions based on the context of real-time analytics. An approach such as Edge Computing allows data to be processed outside the traditional data center or cloud. It is estimated that 75% of enterprise-generated data will be processed at the Edge by 2025. Serverless practices from DataOps toolkits already allow businesses to reduce their amount of hardware and to scale easily and quickly. Almost 50% of companies are already using or plan to use Serverless architecture in the near future.
To wrap it all up, it’s crucial for companies to stay focused and continue digital transformations by adopting novel solutions and to continue to improve the way they work with data so they do not fall behind.
Key contributors
Authors: Marian Faryna, Boris Trofimov
Editors: Liuka Lobarieva, Yana Arbuzova, Den Smyrnov
Special thanks to Iryna Shymko, Olena Marchenko, Alexandra Govorukha, Solomiia Khavshch
DISCLAIMER
The material and information contained in this overview is for general information purposes only. You should not rely upon the material or information in the overview as a basis for making any business, legal, or any other decisions.
Sigma Software makes no representations or warranties of any kind, express or implied about the completeness, accuracy, reliability, suitability, or availability with respect to the overview or the information, products, services, or related graphics contained in the overview for any purpose.
Sigma Software will not be liable for any false, inaccurate, inappropriate, or incomplete information presented in the overview.

Boris Trofimov, a Software Architect with 15 years of experience in software development, architecting, team leading, and educating. Throughout years, he has successfully participated in tons of projects shaping Big Data architecture for companies in diverse industries. His portfolio goes from creating cost-efficient Big Data solutions for innovative startups & getting them delivered in 2 weeks to design of advertisement data platform for the owner of the largest advertising and video platforms with millions of users and billions of video plays. The platform that was developed by Sigma Software team was capable to process 120TB data every day on 2.5M events processed per second.
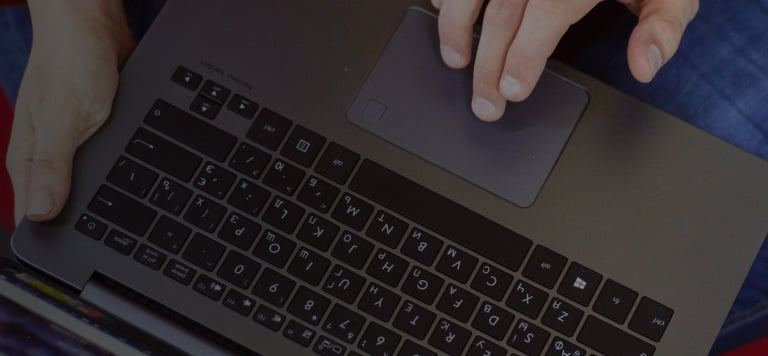
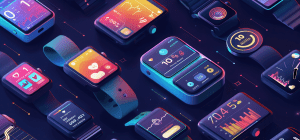
The growing requirements of transplant centers for better post-transplant care have led to wearable technologies becoming an effective solution for patient moni...
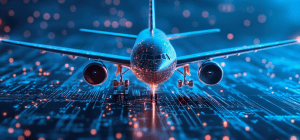
Airlines more often become targets for cyberattacks as technology becomes central to modern air travel. Hence, ensuring robust cybersecurity is now equally as c...
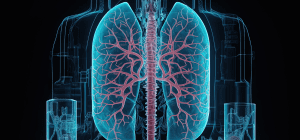
The organ transplantation industry faces major issues regarding patient identification, prediction, and treatment after organ transplantation. In the last few d...