USA
Key Insights on Cost-Saving Approach in Regulation of Bank Data Webinar. Part 1
The Cost-Saving Approach in Regulation of Bank Data webinar by Sigma Software took place on Thursday, May 14th. It was held in partnership with Datrics.ai and Sigma Software Labs and gathered audience interesed in digital transformation in financial institutions. The webinar is one of a series of free webinars to guide businesses on how to continue to move forward during this global pandemic. Our webinars bring together industry leaders from all over the world to provide expert views on how to stay successful while managing the effects of the current crisis.
This time we have invited proven experts to a webinar to share their thoughts on the changes that AI and automation can make in the Banking industry and how a Data Science approach can save costs. Randeep Buttar, founder of Compliance as a Service and a data-led transformation bank expert, and Volodymyr Sofinskyi, co-founder of Datrics.ai, a proficient Data Scientist and Machine Learning Consultant were our speakers. They talked about their hands-on experience of transforming the data-related processes in financial institutions and automating routine banking operations.
The webinar host, Nick Houghton, holds the position of CEO at Think Legal Tech, a Copenhagen-based company that tracks all the innovations happening in the LegalTech, RegTech compliance space, and then helps to bring them to customers.
Nick: We’re here to talk about the regulation of bank data and more specifically, how to manage data regulation, how to save money, and how to unlock value.
Randeep: Good afternoon! My name is Randeep Buttar and I’m the CEO and founder of the company Compliance as a Service Ltd. I’ve been working within the financial services industry for about 15 years primarily as an independent consultant occupying many director type roles in the regulatory change space.
I’ve worked on a number of different initiatives, for example, the EU directive on corporate governance. The directive ensured the presence of the necessary processes for the payments central securities depository to deliver a cross-border platform for payment transfers across Europe. In addition to that, I was also dealing with market risk exposures to highly complex traded products like CoCo bonds.
More recently, my focus has been in the capital markets arena, including regulations like BCBS-239, which was mainly focused on risk data aggregation and risk reporting. What we mean by that is when a bank has to demonstrate how it manages its risk on its balance sheet, how that is being achieved, and how much confidence we have in the underlying data. I think it is one of key topics that we want to unpack here today.
Most recently, I was working for a tier one bank in the Basel III reform space. Basel III is the biggest regulatory framework that has hit the industry since the great financial crisis. Given today’s situation, it’s even more relevant now. The main thrust of Basel III was just to ensure that, from a systemic risk standpoint, banks were well-capitalized. To ensure that we didn’t have to go to citizens to be bailed out and that we had provisions in place if there was a systemic crisis within the industry. Banks must be able to wind themselves up without having too much of an impact on the surrounding infrastructure.
Of course, underpinning all of this is data. Without data, you’re unable to really represent the risk position of your bank and to determine how compliant you are against given regulations. Within that context, I’ve been involved in delivering change, and change itself can be unpacked into many different areas: change in relation to IT Services, management changes related to business continuity, change in relation to things like risk reporting, organizational change, system change, and data change.
My perspective today is in relation to why data is important to regulation and also why that data if used correctly, can be leveraged to deliver operational efficiencies, operational change, and ultimately cost savings. Because right now we’re operating in an environment where the margins are squeezed for banks. They have been since the financial crisis and that’s only gotten worse now. The banks are now being asked to take on a lot more debt and potentially very bad debt – which provides a backstop by governments just to get the economy going as a result of the seizure of the economy in this current pandemic scenario.
I would like to put focus on how to unlock the potential of data to make yourself more competitive and more efficient. Banks can achieve operational gains, but they can also become more competitive in the marketplace, because the underlying data sets are the same.
Volodymyr: I’ve been working for a London top tier investment bank in their back office for roughly three years doing mainly a quantitative analysis and data analysis job, working with Basel III as well. After that, I switched to purely data science, basically managing a team for almost three years.
Now we have started Datrics.ai, which is a platform for easy data science. It’s a platform that simplifies the MLOps a lot more and lowers the entrance barrier for data scientists as well as for practitioners.
Nowadays, retail and banking are the top two industries in AI adoption. Artificial Intelligence is set to save over 1 trillion dollars by the end of the decade for the banking industry alone, while the latter is expected to spend around 5 billion dollars on the implementation of AI solutions over the next two years.
The data itself can be used in various ways, not only for regulations. You can monetize the data or decrease your costs right away. If your company or bank is gathering a lot of data, you can use it to decrease your costs, to target your customers better, or to optimize your operations and bring additional value in this way.
The biggest challenge with banks is that many of them are using old legacy systems that are very big and very difficult to modify. Some are in COBALT, some are in C or C++, and all of them are usually quite difficult to work with. This is why platforms that can interact with your stored data, create a data science pipeline separately, and easily integrate at the very end with your existing systems, are on the rise right now. It’s very important because across the industry and generally in data science, more than half of the projects that do data science never go to production.
The big problem with data science is that first you have a lot of data and makes you wonder “hey, can I actually use this? Does it have predictive power? will it even potentially save me money?” You would start with something like a feasibility study or a proof of concept to have really nice results. So, you allocate some resources to start building the product and somewhere in the middle you understand that the proof of concept does not scale very well in production. For example, running a daily model and a proof of concept is nice, but if that model on your full data set runs for three days you cannot run that daily.
This is why I like having a unified tool that allows you to do prototyping and use feasibility studies. A tool that scales up to any amount of data and integrates easily with your current infrastructure. The problem usually is that a lot of data scientists in the market don’t know too much about banking and a lot of people with experience in banking don’t know too much about data science. Basically, you can either try to find a person who is like a “jack-of-all-trades” but usually it’s going to be either very costly or a “jack of all trades and master of none.” This is also why the platforms are quite useful because banks usually have their own very nice analytic departments that just don’t have too much experience with data science. Or, for example, the analysts don’t have enough experience in writing code. If you had a platform that would allow them to do data science related jobs without writing the code that might help a lot. The good news is that we see platforms of these types are on the rise right now.
Nick: One of the challenges we always have with these types of things is explaining it to the rest of our colleagues that don’t necessarily understand what it is we’re doing. So how do we show them some value as quickly as possible? Does a proof of concept help with this? Why should companies and banks invest time and resources in this?
Randeep: One of the roles that I occupied quite recently was as the head of data strategy and innovation. With my innovation hat on, we went out to our organization and started polling them for use cases. I was fortunate because I was working within an environment where innovation was encouraged extensively. The head of our risk transformation department was very supportive and helped create a framework within which you could actually contribute ideas in a centralized hub and almost crowdsource those ideas. From time to time the top five ideas would be selected for a proof of concept.
About 90% of the ideas are going to fail, but that’s okay. You have the freedom to fail as long as you try. The key thing was to really be able to demonstrate the value in the proposition and what you were trying to do.
Now there are so many different types of innovations that are gripping banks at the moment. Repetitive process automation (RPA) is one of them. Essentially, it’s the use of bots to automate basic activities. For example, you might have credit analysts that goes in every day, take a dump of data of say, Bloomberg, download it, extract it, paste it into a CSV file, map it against some internal data, run some macros, and essentially end up with a result that they have to email a bunch of people. That could take up to 45 minutes to an hour every single day of this person’s life.
To automate this process using our RPA, you need just a couple of minutes – screen scrape and run the macros. The whole thing is repetitive, and the tools are now becoming available which make things a lot easier. I like to cite this concept. We call it citizen development, whereby somebody who’s not a coder is able to use drag or drop techniques to just throw it into an interface and generate code. That is empowering in itself.
I currently function in an environment where my particular bank has operations in 66 countries. They’ve got about 39 million customers and about two hundred and ten thousand employees. They’re very vast, very complex, it’s the other end of the scale. To be successful in an environment like that, not only do you have to prove yourself out in a local manner, but you also have to demonstrate the ability to scale across a multitude of complex environments.
System integration is key. This is important for many banks, especially the European ones. One of the reasons why they had problems in the last financial crisis was because they had evolved over decades through mergers and acquisitions. They’d accumulated a lot of systems from the banks that they had absorbed. However, they weren’t so great at cross-functional integration or cross-system integration, so they ended up with this quagmire of systems landscapes. We call it “technical debt.” It is okay if you’re in a straight-through processing siloed environment, and the systems can handle it. But as I mentioned earlier, we’re in an environment which is essentially low in volume, and margins are being squeezed. Because of that, you need more cross-functional integration across a business or functional lines for all areas – private banks, commercial banks, retail banks, finance risks, etc. You need to be highly integrated to gain some form of efficiency. So whatever you’re trying to do, it has to be Agile, it has to be nimble, and it has to work across that environment.
Nick: Volodymyr, do you have any examples of this?
Volodymyr: One of the things is automation. While I was working in investment banking, quite a few analysts were just drilling down into the code and figuring out how the calculations work. Because of PRA requirements, many algorithms need to be upgraded right away. In the big banks we were calculating over three and a half million of trades worth of NPV. I believe that would be around fifty years in the future and we were running Monte Carlo, so that was quite a lengthy process. One batch run would take around 24 hours. The problem with that is if you are upgrading your system on a weekend it might be problematic. If you have some delays, on Monday you cannot start. We implemented a simple AI for that – we had a health status dashboard pretty much just parsing the logs and trying to do some anomaly detection and root cause analysis for what went wrong.
It made things very easy. Before, if ever the director didn’t like the numbers and told the analyst to drill into them, the analyst could spend 2 to 8 hours just figuring out which of the trades overshot the NPV. We automated that to the extent to where anybody could figure out which specific trade or algorithm was breaking the threshold or looked like an anomaly. Instead of trying to find the root cause, the analyst looked at the algorithm and tried to figure out where the calculations were wrong. The cool thing about AI is that you can automate a lot of stuff, but the best thing is that you can automate routine tasks.
Nick: We see this all the time in legal where everybody talks about wanting to automate what happens in the courtroom. Let’s start with the really basic stuff of sifting through the kilometers of documents that go into a major file and major court cases. You’re automating the basics, giving people the knowledge of where to look, and which question to ask. It is way more important than just having the right answer. Getting the right answer is just a matter of time, but you can save a huge amount of time if you know where to look. Can you give some examples of this?
Volodymyr: Automating the help desk job for example. After some analysis, it turned out that in around 60 to 70% of the help desk requests, a person couldn’t connect to a printer or forgot their password. Those two things can be easily automated; we just created a chatbot to automatically assign tickets. If a ticket didn’t need manual intervention, we just triggered some partial scripts and automated everything. The main result was a decreased response time for critical issues. As for the people who used to call support and spend five minutes trying to reset their password, with this automation they got their problem solved almost instantaneously.
Nick: Haley, a junior employee working in the front office, just wrote: “I’m in sales and product management, and am out of reach with the innovation team. So how can people at the front with the problems get a hold of people like you?” A super question, office teams in a bank can help a digital transformation process, but front and back offices are often disconnected.
Randeep: A lot of it is down to the prevailing culture. From my perspective, people like Haley are absolutely gold. We’re on the hunt for SMEs and we’re looking for people who are on the front lines, who understand the business, who understand the nature of the actual products themselves. Therefore, these people are our best place to get recommendations. Leave the tech stuff to the tech guys, but what we need from you are your ideas and your thoughts around how we can improve our processes and do it in a way that’s going to be generally beneficial.
Nick: Oftentimes, it’s not what the solution is but it’s explaining what the problem is. I have an example of this. We had a new piece of technology that was an upgrade and it replaced a simple process. When I had a question, I pressed a button, and I got an answer. This process was replaced with a twelve-step flow that took half an hour. Instead of five seconds and one button, it came to 30 minutes and 12 steps. Until I wrote all of this down in an email, which took me an hour, nobody around me understood the problem. They thought it was an upgrade, but actually it made my life much more complicated.
Randeep: You’ve just hit on the key point there. I think the answer for Haley here would be to come up with a very clear and crisp articulation of what the problem is and to define the problem statement. It’s almost like a mini business case, so you want the description to be short, sharp, and punchy, and explain to management that we really do have an operational problem here. You don’t necessarily have to identify the solution, because ultimately it’s up to them to come up with one. They need to throw resources at your problem to refine the business case.
I think that really is the future for employees – to project their mind into the direction of automation. If they’re involved in a repetitive activity in their everyday job, they can almost guarantee that this job probably won’t exist in a couple of years’ time. A lot of us have to re-skill right now and start focusing on the things that are actually going to bring value rather than just repetitive activity. If you are able to articulate that to your management team, that’s probably the starting point. Come up with that mini business case, outline the benefit that can be broken down into a number of dimensions: a cost-benefit, an effort benefit, a product benefit, or a benefit to the customer. The customer is always top on the list for everyone, so it’s a good idea to focus on how automation will benefit them.
If you look at some of the banks out there, in the Near East, they can approve or reject a loan request super-fast. We’re talking a matter of hours, in some cases even minutes, because it’s all automated now, including credit checks, gathering information, etc. A lot of banks in the West are still running quite a lengthy process to get through that cycle. Therefore, you will get a lot more traction if you can demonstrate how this will give your organization the ability to benefit its core customer set.
End of Part 1
Soon in Part 2 of Key Insights on Cost-Saving Approach to Regulation of Bank Data Webinar – data quality criteria, establishing a complete framework for data management and data governance, and real-life examples of automation in Banking.
We hope that the insights that our speakers shared will help you bring more automation into your organization and introduce healthy data gathering and analysis procedures. We will keep bringing you outstanding experts that are transforming businesses in various domains. Follow us on LinkedIn, Facebook, and Twitter to stay updated on our news and events.

Sigma Software Group provides IT services to enterprises, software product houses, and startups. Working since 2002, we have build deep domain knowledge in AdTech, automotive, aviation, gaming industry, telecom, e-learning, FinTech, PropTech. We constantly work to enrich our expertise with machine learning, cybersecurity, AR/VR, IoT, and other technologies. Here we share insights into tech news, software engineering tips, business methods, and company life.
Linkedin profile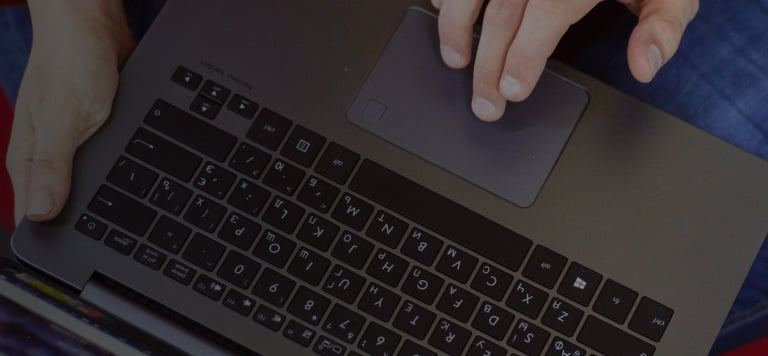
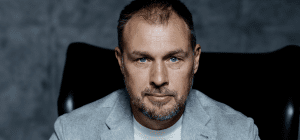
Valery Krasovsky holds the position of CEO at Sigma Software for over 10 years, switching from the position of Chief Operating Officer in the same company. Duri...
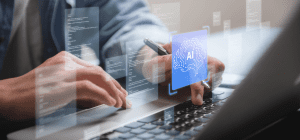
We are thrilled to announce that Sigma Software has officially been recognized as an AI Data Cloud Service Partner with Select Tier status by Snowflake! This is...
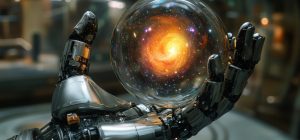
Sigma Software together with our corporate venture wing Sigma Software Labs launches Labs Galaxy - an internal community of innovators. Focused on ideas discuss...