USA
Key Insights on Cost-Saving Approach in Regulation of Bank Data Webinar. Part 2
This April, Sigma Software introduced a series of free webinars to guide businesses on how to continue to move forward during this global pandemic. Our webinars bring together industry leaders from all over the world to provide expert views on how to stay successful while managing the effects of the current crisis
Our second webinar, Cost-Saving Approach in Regulation of Bank Data, took place on Thursday, May 14th. We welcomed experienced tech specialists – Randeep Buttar, founder of Compliance as a Service and a data-led transformation bank expert, and Volodymyr Sofinskyi, co-founder of Datrics.ai, proficient Data Scientist and Machine Learning Consultant. They shared their ideas on how to effectively implement a Data Science approach and save costs.
The webinar host was Nicholas Hawtin, CEO of Think Legal Tech, a Copenhagen based company that tracks all the innovations happening in the LegalTech RegTech compliance space and then helps to bring that to customers.
You can find the first part of the webinar discussion here.
Continued:
Nick: One of the concerns spreading across the Banking industry is whether your data is good enough and, whether you have enough of it. How do we ever get all these data sets aligned? While oftentimes you don’t have to have perfect data, you just have to have enough data to be able to do a couple of checks. You can run checks across different data sets and, you don’t have to pool everything into one huge lake and have one ring to rule them all. We can do checks back and forth between them to give us valuable information.
Volodymyr: That’s how all data science and all statistics in general work. If you are making soup, one spoonful is enough to taste it. You don’t need to eat the whole pot. It’s the same with data. If you have your soup stirred properly, it means you can sample a very tiny portion of it. If your data is representative of the big pool you have, you can work with a very little part of it. You can do a feasibility study or an A/B test. For an A/B test, you don’t need to split all your customers into two, you can split them into 20 different segments and work with each of them in parallel. You don’t need to work with everyone to be statistically and significantly sure that all your calculations are correct.
Randeep: So we’re talking about sample setting the data essentially. One of the areas that I’m really interested in at the moment is graph stores. Most people, when they approach data historically, if you think about the way in which typical old school reference data are set up, they’re highly structured by primary key, foreign key joins, and that sort of thing.
Some of you may remember the Panama Papers story where a group of journalists read across about 30 terabytes of unstructured data in PDFs, Word documents, and Excel files. They were able to make inferences and conclusions from that vast dataset within a matter of months using graph technology.
Essentially graph technology disambiguates the entire data set and makes every single bit of data foreign key. As long as you can create a dictionary that allows you to describe what each element is and then you can start linking things up in a way that you never could before. As long as you define the relationship from one entity to the other, you can read across a multitude of datasets and just get inferences that you never would have been able to achieve or would have taken you a very long time to achieve historically.
Nick: People often worry about having enough data and are concerned about their data not looking fantastic. How about we take data as our first challenge. Can we say how much data people need to have access to and how clean it needs to be in order to draw conclusions from it?
Randeep: One of the things I was asked to do a couple of years ago was actually help a new chief data officer establish a complete framework for data management and data governance. It was very much about getting back to basics. The first sorts of questions you might ask are “What is the data? What data am I actually looking at here?”
Some of the recent fads in this area are data lakes and getting everything into the cloud. However, if your data lake has got a bunch of unidentifiable data, it’s effectively a data swamp. And if you just literally lift and shift that to the cloud, you’re recreating the swamp in the cloud. So cloud is not a cost saver, it’s more of an enabler for better analytics.
I’d say there are four steps to establish a complete framework for data management and data governance.
Step one is be able to identify your data, make sure your data is linked up to a good conceptual data model and has got definitions within that hierarchy. Definitions are your linchpin and your starting point. Beyond that you can go to where you should be getting your data from. As an organization you don’t want to be getting the same data from multiple places and multiple vendors. You can incur costs as a result of that. In order to reduce your costs, you should standardize your golden sources of data and make sure that any specific types of data are coming from one designated distributor.
Step two is data flow and lineage. It relates to the questions “Where is my data coming from? Where is it going to? How many hops are along the journey? Is there an efficiency opportunity there?” You may look for cases when data is just circling around a number of loops unnecessarily or going off flying into somebody’s own developed application within Excel or access stored on their desktop, which becomes a single point of failure. You can spotlight those issues, rip them out of your flow, and clean things up a little bit.
The third step is quality. In order for you to do all the great stuff that Datrics are doing in the data science space, you need to make sure that you’ve got good control around your data and your data is of a good enough quality. At the end of the day, AI is as good as the data going into it. It’s garbage in, garbage out. Your data must be of good quality – accurate, coming from the right place, produced in a timely fashion, not rolling forward previous runs of data, and complete. Ensuring all of that needs to be part of your data management lifecycle as well as other things like basic data validity, formatting rules, checks, un-checks, etc.
Once you’ve got those three steps in play, you can move forward knowing that you can believe in the data that you’re working with. It becomes very important when you’re producing reports for the regulator and/or senior management.
The fourth step is a senior manager certification regime. Here, accountability is being driven on a personal basis. At this step, you need to work on the data cleansing and data management space. Once you build those foundations, you can continue with data science.
Nick: So, if we want to do a proof of concept, how small can this proof of concept be? If, let’s say I’m looking for anomalies, how small can my dataset be if I’m looking to get it to a trial?
Volodymyr: Once we did a very small proof of concept where we were given a few hundred megabytes of data. That’s a tiny amount of data that you can work with on a home computer. The actual data set was over one terabyte so our amount of working data could be less than 1% of the whole data set. With that amount we were able to figure out how much money we could potentially save and statistically calculate it for the whole data set.
Here is a small example from my experience. We had a customer from the retail industry. The customer was interested in automating the work of the inventory managers who were responsible for each stores’ purchasing and stock. Their data was very nicely structured and easy to work with; and what actually happened is that instead of just automating the replenishment process, we optimized their entire inventory management. It turned out that a significant part of their capital was allocated to stock. Through inventory management optimization, we decreased the average cost of inventory by around 40%. For some non-critical items we even managed to decrease the stock by 70 to 80 percent. What helped in this case was a look at the data from a different perspective and with an understanding of how to utilize the data.
Nick: When we talk about the supermarket industry, we get excited at the possibility of a 2 or 3 percent improvement. So when you mention 40% it seems like a pretty crazy number and people in logistics get extremely excited.
Volodymyr: That was a very big construction company in the States. The store managers only felt confident with piles of building materials lying in the yard. However, you can optimize that quite a bit and people will still visit your shop.
Nick: What could you do with 40% savings in terms of data science?
Volodymyr: One agency comes to my mind right away. They were doing a ton of promotion campaigns, performing A/B testing, and gathering a lot of data. We offered to feed the data into a machine learning algorithm and have promotional campaigns generated by the algorithm instead of having a person thinking about which is the best approach. With an algorithm, they could perform 10 different promotion campaigns instead of A/B testing. It allowed them to do A thorough F testing and figure out what is actually the best option with the most significant savings.
Nick: This sounds nice but how do you start with machine learning?
Volodymyr: Machine learning on the conceptual level is quite easy; you just feed it a lot of data. Then you define the success criteria. For example, if you are trying to figure out how many people respond to a promotional campaign, you define this value as the success criteria and try to maximize it.
Machine learning algorithms usually don’t care what the actual meaning of the data is. You give it the data and the machine learning algorithm determines that a certain combination of data is the best for your success. Then a person with good subject matter expertise looks at those numbers and chooses which of the algorithm results makes sense and which don’t.
Randeep: Let the machines make the inferences, but then humans will always be required to validate whether or not those inferences are correct. I think that’s where most people need to be shifting their skillsets towards – becoming the subject matter expert that can help the machine learn. You can throw as much data as you want at the machine but it’s only going to be as good as the inferences that give you a high level of probability that the data is matched correctly.
Nick: Do we have any tips for where to look to find low-hanging fruit in RegTech or compliance?
Randeep: Compliance is probably the last bastion of manual processes within any financial institution. Other areas have been on their journey towards automation for quite some time with heightened data analytics and the rest of it. There’s a lot of low-hanging fruit all over compliance right now. All you’ve got to do is grab the compliance analyst and they’ll probably tell you a tale of woe about the amount of manual work they need to do repetitively. They have to keep track of a constantly changing landscape and to do that manually in this environment is just impossible. The key trends within the industry are that you’re getting a lot of regulatory scrutiny, but the volume of regulation is going through the roof. It’s not abating and you’ve got multiple regulators asking for similar things in different ways. So you absolutely need the support of technology to help drive through it.
A big low-hanging fruit opportunity is optimization and identifying areas where an efficient operational process is absent. Answer the question: where do you have a duplication of feeds? Where do you have similar systems doing the same things or even multiple vendors that you’re paying for similar services?
A starting point is to have good metadata, which describes your estate. Then use it to understand where your hotspots are. For example, it could be a multitude of vendors, end-user computing that’s taking up a lot of hours of work, a multitude of feeds, etc. There’s a whole host of inefficiencies all throughout, not just in compliance but in any organization.
Nick: I work a lot with entrepreneurs across the spectrum. One of the pieces of advice I could give to young people looking at getting into MedTech or health is to say: “Walk into a hospital, look for somebody that’s got a pen or a pencil in their pocket and ask what they do with that pencil, and then automate it.”
There are similar things to do in other industries. Every organization has dozens of manual things happening every day. Look at them, grab them, and then have that person do something else with their life.
Randeep: I agree, it’s all about empowering people.
Nick: Absolutely. Volodymyr, any last thoughts here as we approach the end?
Volodymyr: I would like to encourage people to play with data science in general. It’s a very beautiful field of science and it probably makes you look at some things in a different way and you will start to see patterns that you didn’t see before.
We hope that the insights that our speakers shared will help you bring more automation into your organization and introduce healthy data gathering and analysis procedures. We will keep bringing you outstanding experts that are transforming businesses in various domains. Follow us on LinkedIn, Facebook, and Twitter to stay updated about our news and events.

Sigma Software provides IT services to enterprises, software product houses, and startups. Working since 2002, we have build deep domain knowledge in AdTech, automotive, aviation, gaming industry, telecom, e-learning, FinTech, PropTech.We constantly work to enrich our expertise with machine learning, cybersecurity, AR/VR, IoT, and other technologies. Here we share insights into tech news, software engineering tips, business methods, and company life.
Linkedin profile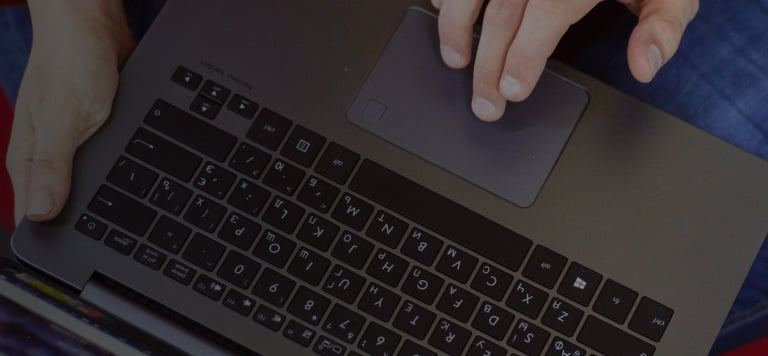
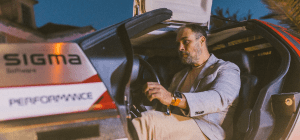
Ten years ago, in December, Valery Krasovsky became the new CEO of Sigma Software, switching from the position of Chief Operating Officer. Over the years that p...
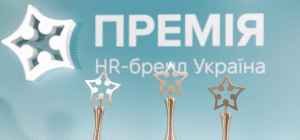
Sigma Software is committed to removing barriers based on bias, not only within the company but in the industry in general. So, we decided to highlight this dir...
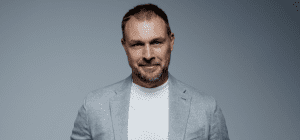
Valery Krasovsky, CEO and Co-founder of Sigma Software Group talked to Forbes.ua about adapting the business to war conditions and responding to the AI boom. As...