USA
Prompt Engineering and Efficient Work with AI
Content creation, game development, customer engagement, language learning, medical research, prototyping, code generation – these are a few new areas that GenAI will influence in the near future by enhancing creativity, automating complex tasks, and enabling new possibilities. We, at Sigma Software, believe that change is a natural part of life and try to embrace it, not resist. So, we discussed with Max Kovtun, Chief Innovation Officer at Sigma Software, how to get maximum efficiency from generative AI models through the quality of input we provide—a concept known as prompt engineering.
Prompt engineering involves crafting precise, context-aware instructions that guide AI to produce accurate and valuable outputs. Mastering this skill ensures better outcomes, reduces errors, and unlocks the full potential of these advanced systems. In the AI-driven era that unfolds in front of our eyes, prompt engineering is not just a technical skill but a vital bridge between human creativity and machine intelligence.
Max Kovtun is not just an experienced software architect, he is an enthusiastic innovator always eager to try every new technology that hits the market. With AI, he implemented in the company an internal GenAI service called AI Assistant to enable Sigma Software specialists to get all the benefits of tools like ChatGPT but in a secure way, suitable to work with sensitive data. Max also introduced additional features to AI Assistant, like drawing graphics and performing routine tasks – sending emails or creating meetings in the calendar.
– What would you call the main components of a well-written prompt? What should a query contain if a user wants to get an accurate answer from a chatbot?
Nothing in our world is objective – we perceive everything through the prism of our experience, knowledge, qualifications, and current situation. When we communicate with a person, we are in a certain context, we know something about each other, we can make some assumptions about what the person is asking or telling us and why. We can also clarify something, for example, ask “Why are you asking about this particular indicator? What problem are you currently trying to solve with this information?” The common context brings the prisms of perception of the interlocutors closer together and makes our communication more meaningful and more effective.
It’s important to understand that when we open a new chat with an AI tool and type in a question, it doesn’t have any of this. It doesn’t know who we are, what problem we’re trying to solve. It has no context to make assumptions about what’s important, from what perspective to consider the question, what information will help and what information will be useless. Therefore, the first thing you should pay attention to is describing your context and the grounds of your question.
Have you provided enough information for the model to understand who you are, why you are writing this prompt, what task you are working on, and what criteria you use to evaluate the quality of the result? Use the techniques of setting tasks for your subordinates to formulate a good request for the model.
– What happens if you don’t provide enough information in your request?
The LLM will try to fulfill the task and may find connections or meanings that you would not have considered appropriate. For example, here is a query in which the author asked to describe the content of a book and somehow connect it to the fact that the description will be provided to our employees working in Ukraine.
The model made the connection, and it may look like there are references to Ukraine in the book, although there are not, and the connection that the model found is weak.
ChatGPT answers: You are correct, and I apologize for any possible confusion. “Trump and the Post-Truth World” by Ken Wilber focuses primarily on cultural and philosophical aspects related to the 2016 U.S. presidential election and the rise of post-truth culture. The book does not directly mention Ukraine. My previous message aimed to connect the book’s themes with our global context, including our colleagues in Ukraine. I apologize again for the misunderstanding.
Therefore, it is important to question the information and statements contained in the model’s response. Use critical thinking approaches and techniques to validate the information received from the model (and, in general, from anyone).
– How would you describe the approach you use in composing prompts?
Communication between people is based on interaction. We rarely need to clearly and completely formulate a request to another person and consider all possible uncertainties.
In such situations, we spend a lot of energy to make sure that we have asked everything we need to, that the questions are formulated clearly and unambiguously, etc. We will prepare these questions the way you need to write a one-shot prompt for an LLM.
But this is an exception, and all other communication between people is iterative – we formulate questions of “sufficient quality in our opinion” and look at the interlocutor’s reaction. When we see that the other person has misunderstood something, we provide clarification. When we see that they provide us with information that we think is irrelevant, we ask how this information is related to our request.
– What is the most common mistake that users make when communicating with tools like ChatGPT?
That is, if we haven’t spent an hour formulating a tender-style query, we shouldn’t expect to get a clear, comprehensive, and super useful answer the first time.
Instead, we should use the interactive approach we are used to – provide a piece of information, look for signs in the answer that the model has misunderstood something, provide more information, etc. Talk to it as if it were an intern and plan to get what you want as a result of the dialog, not from the first reply.
– Any other tips regarding communication with LLM?
Models learn from texts written mostly in English, so it makes sense to communicate with the model in English until you get a satisfactory result, and then ask the model to translate this result into the desired language.
– What the prompt must not include if the user wants to get an informative answer?
The approach should be the same as when setting a task for a subordinate. If you provide them with information that is not necessary to complete the task, it can confuse them.

Sigma Software Group provides IT services to enterprises, software product houses, and startups. Working since 2002, we have build deep domain knowledge in AdTech, automotive, aviation, gaming industry, telecom, e-learning, FinTech, PropTech. We constantly work to enrich our expertise with machine learning, cybersecurity, AR/VR, IoT, and other technologies. Here we share insights into tech news, software engineering tips, business methods, and company life.
Linkedin profile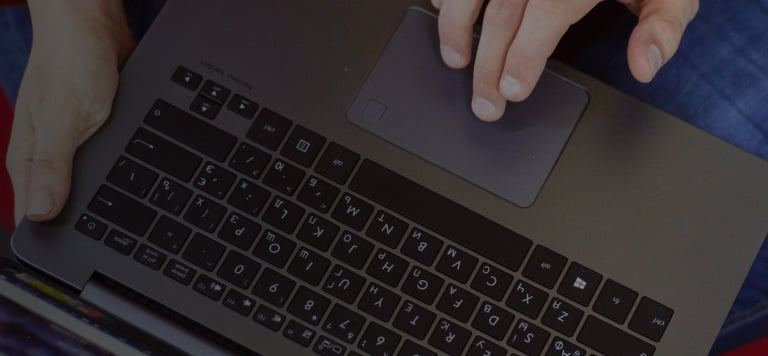
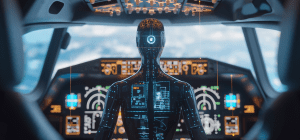
Oleksandr Plyska, Vice President at Sigma Software, leads a global business unit specializing in several industries, including aviation. The unit’s capabilities...
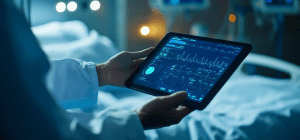
This in-depth guide on real-time patient monitoring platforms covers the main benefits for transplant centers, presents a list of key features for such platform...
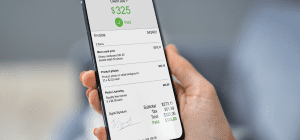
Every billing error isn’t just a technical issue — it’s a hit to your bottom line, a risk to your reputation, and a threat to patient trust. This guide breaks d...