USA
Procurement Planning Software Development
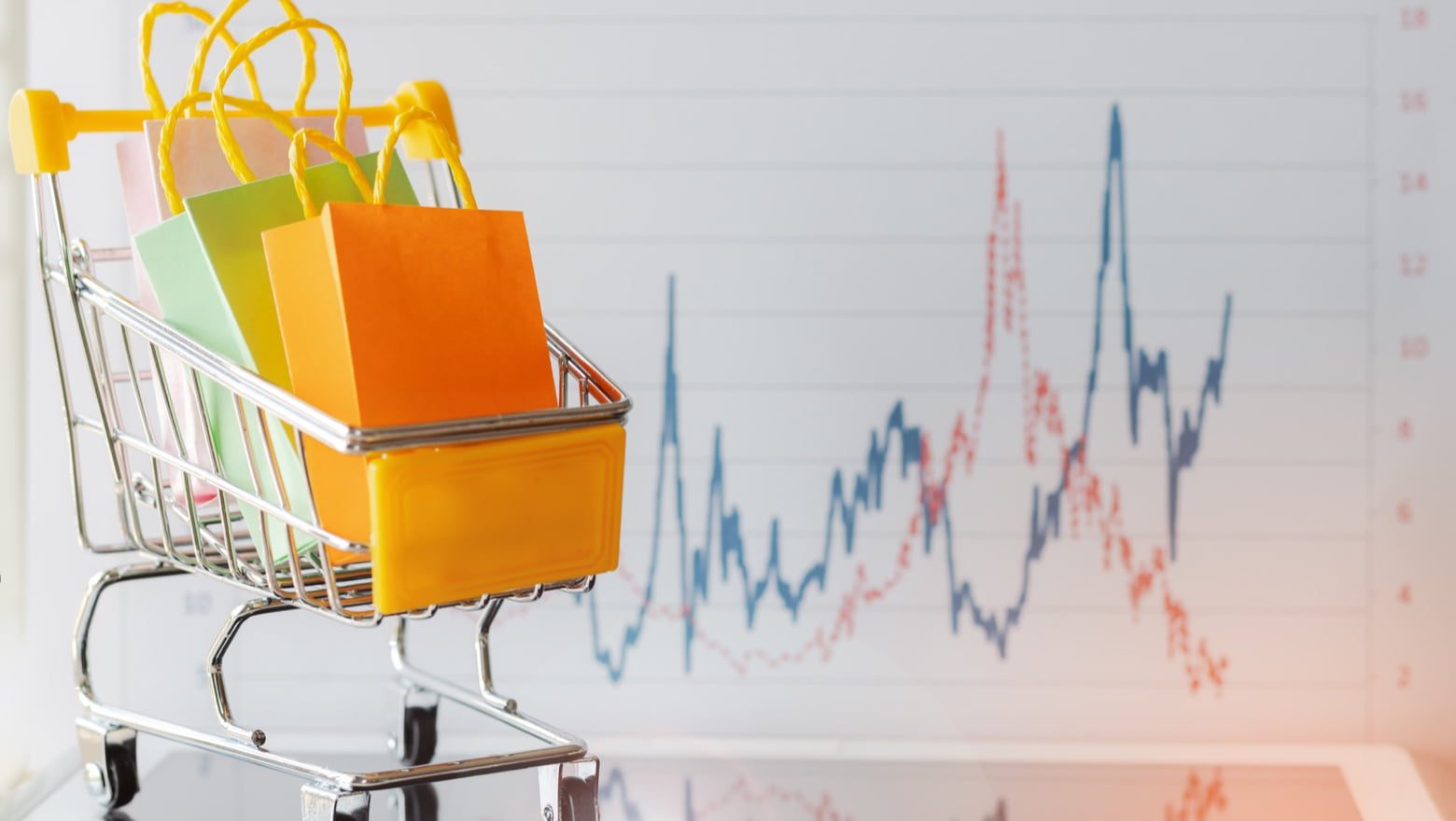
A solution to help retailers, caterers, and others plan procurement of perishable products based on a composition of simple adaptive models. Due to accurate prediction of required amounts of products to be bought, loss resulting from self-life expiration and loss in profit can be avoided.
Solution
Existing procurement planning has insufficient accuracy of about 70%. With this level of accuracy, retailers, caterers, and others dealing with perishable products may buy an excessive or insufficient amounts of certain products. In such cases, they suffer a loss when products self-life expires or when they are out of stock and miss profit.
To address this problem, the Sigma Software team for developing Machine Learning solutions came up with an idea of a procurement planning solution based on a hypothesis stating that:
- Sales of products of different categories vary and should be described with different mathematical models, and
- Mathematical model predicting sales of a particular product category can change irregularly.
The team has implemented the procurement planning solution based on a large volume of historical sales data. To predict sales of different categories of products accurately, the solution was built as an adaptive composition of simple adaptive models. In other words, several different mathematical prediction models predict sales, and the solution uses the model that demonstrated the highest accuracy for the last week. To avoid overfitting, an optimum amount of models is evaluated with the Combinatorial Theory of Overfitting.
The developed solution was tested on real-life data and proved successful. The hypothesis was proved, and sales prediction accuracy improved by more than 10%. As a result of improved accuracy, the loss caused by incorrect procurements decreased. Moreover, since the solution used simple mathematical models for predictions, requirements for hardware resources have also decreased.
Result
The procurement planning solution improves sales prediction accuracy by more than 10% and imposes lower resource requirements.
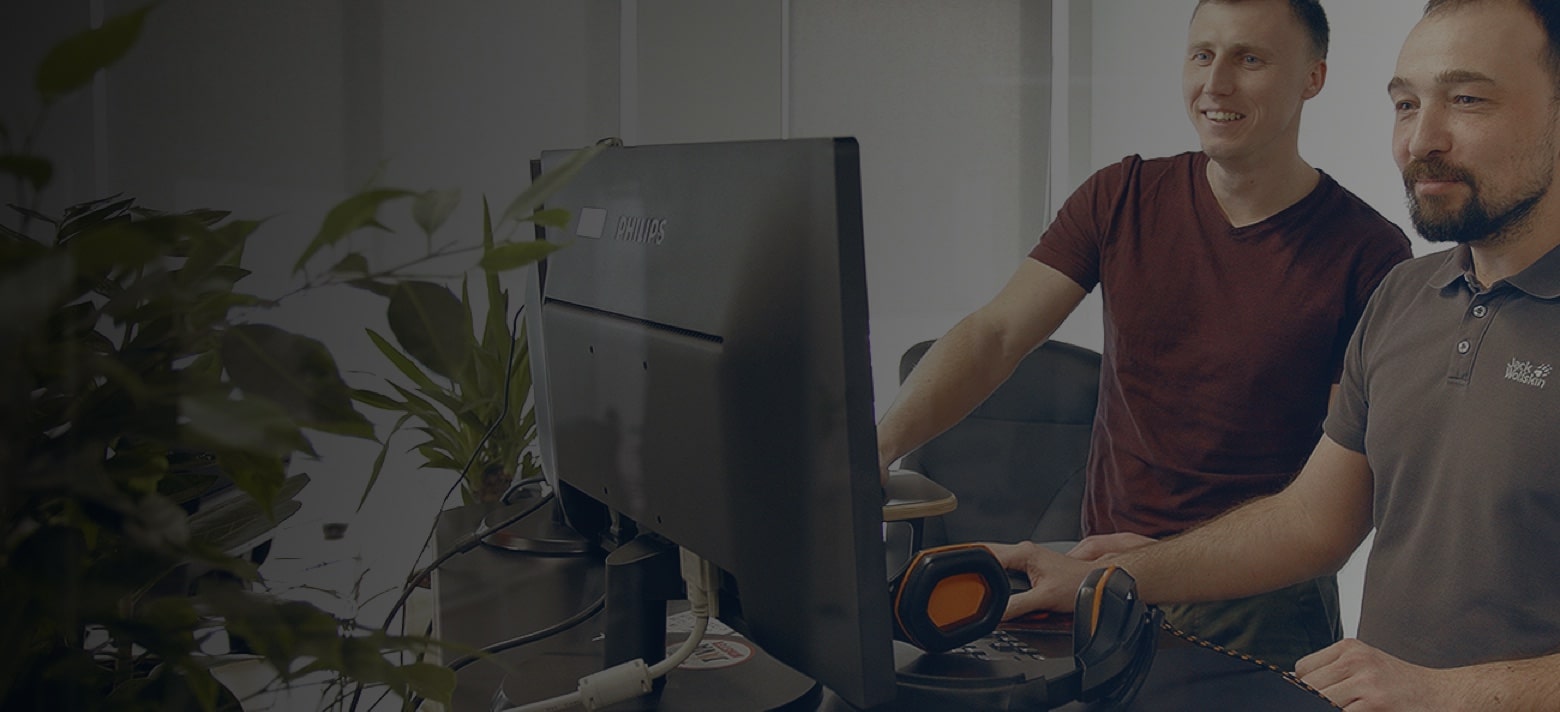
In-house solution developed on the basis of the company Machine Learning experts group
A procurement planning solution to predict sales of different categories of perishable products accurately
Full-cycle development from a concept to the final solution and integration into the existing company infrastructure and with other information systems, including ERP system, BI solution, procurement planning system, and more
Project duration is 3 months with an average of 3 FTE
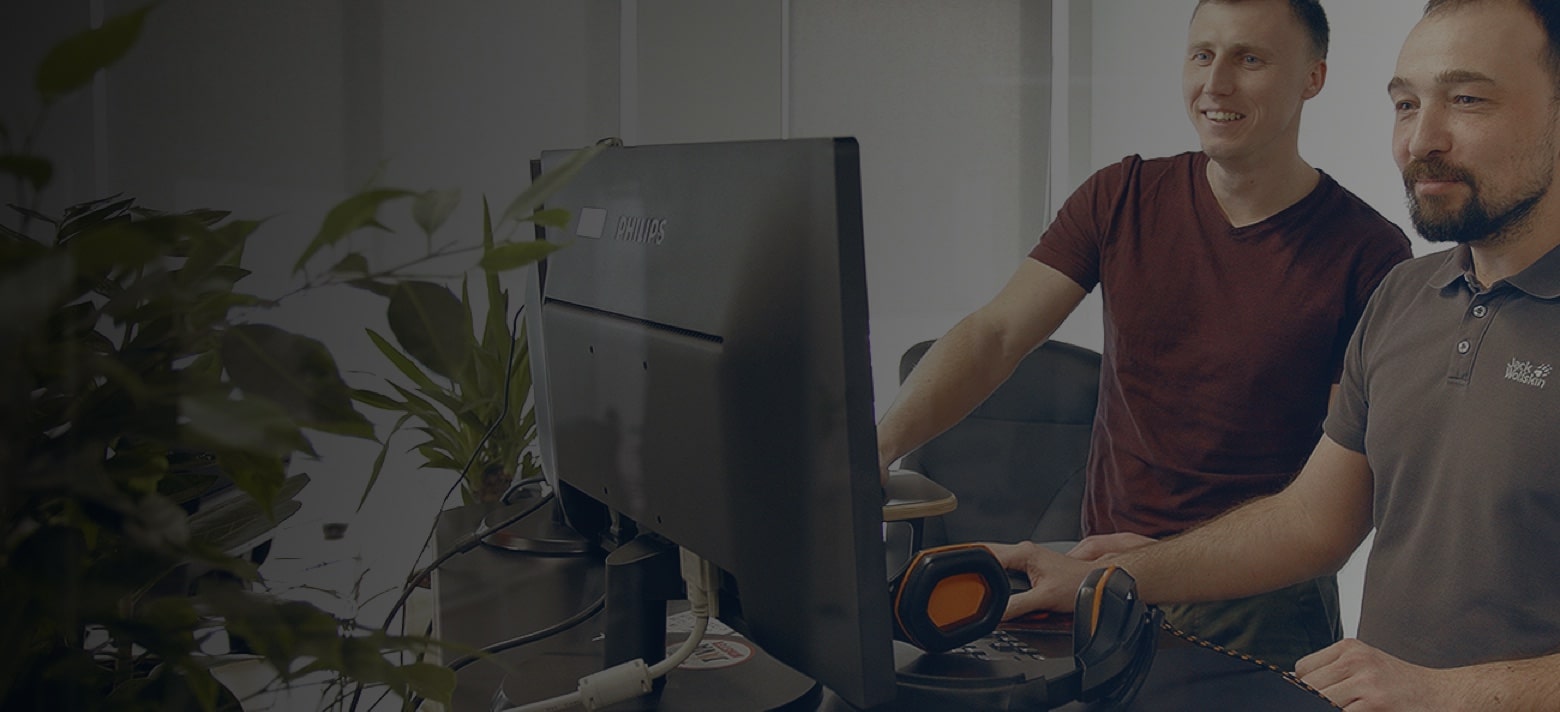